Second prize for this year’s 2023 IoP CPG Thesis Prize has been awarded to Dimitrios Bachtis, Swansea University. Dimitrios’s thesis, titled Quantum field-theoretic machine learning and the renormalization group, explores the derivation of neural networks from quantum field theories and utilizes machine learning techniques to study phase transitions.
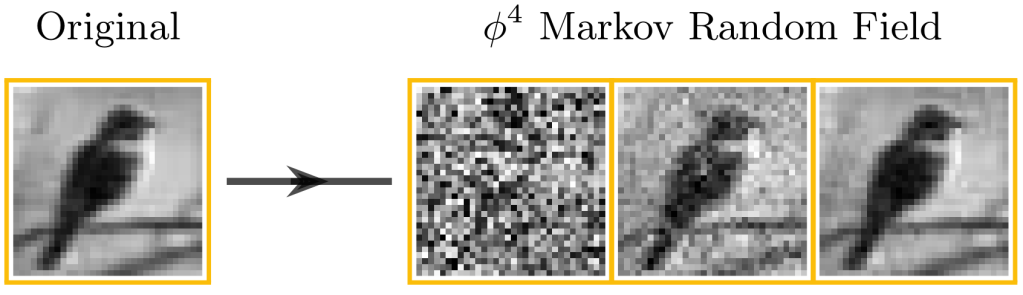
An indispensable tool in the study of phase transitions is the renormalization group, which investigates how a system changes when viewed at different scales. The application of a renormalization group transformation can be intuitively understood as the “zooming out” of a map: as the image becomes smaller some of the fine details within the map have disappeared. Dimitrios explored how machine learning algorithms enable an approximate inversion of the renormalization group which, analogously to the previous example, can be understood as the “zooming in” where new fine details are now introduced by the machine learning algorithm. The method opens up the opportunity to conduct high precision computational studies of phase transitions. Dimitrios additionally investigated the derivation of neural networks from quantum field theories via the use of the Hammersley-Clifford theorem, thus establishing a mathematically rigorous connection between quantum field theory, machine learning, and probability theory.
We look forward to reading more about Dimitrios’ work in the next IoP Computational Physics Group Newsletter. In the meantime, Dimitrios’ thesis is available online.